The recent wave of achievements in artificial intelligence has understandably generated tremendous enthusiasm and optimism. From language models like ChatGPT that can engage in freeform dialogue to image generators like DALL-E that can create novel visual content from text descriptions, AI’s capabilities are expanding rapidly. However, amid the torrent of media hype, it’s crucial to maintain a sober, historical perspective on the repeated cycle of inflated expectations followed by periods of disillusionment that have plagued the AI field.
These “AI winters” refer to times when funding was slashed, companies went out of business, and research stagnated after the lofty promises of AI failed to materialize. The first such winter struck in the 1960s and 1970s. Following pioneering work in areas like machine learning and neural networks, there was a surge of optimism that human-level artificial general intelligence (AGI) was just around the corner. However, the technological shortcomings of the era proved unavoidable. Overhyped capabilities like fully automated machine translation between natural languages fell drastically short of expectations.
The First AI Winter
The first AI winter of the 1970s was largely influenced by the unrealistic expectations and overhyping of early AI research, particularly in the fields of machine translation and neural networks.
The early successes in machine translation, such as the IBM-Georgetown experiment in 1954, led to exaggerated claims in the media about the capabilities of these systems. However, the actual demonstration involved translating only 49 Russian sentences into English with a limited vocabulary of 250 words. Researchers had underestimated the complexity of word-sense disambiguation and the need for common-sense knowledge in translation. By 1966, the Automatic Language Processing Advisory Committee (ALPAC) concluded that machine translation was more expensive, less accurate, and slower than human translation, leading to a significant reduction in funding and research in this area.
Similarly, early neural networks, such as perceptrons, failed to deliver the promised results. The 1969 book “Perceptrons” by Marvin Minsky and Seymour Papert emphasized the limitations of these networks, and the lack of knowledge on how to train multilayered perceptrons led to a decline in interest and funding for neural network approaches in the 1970s and early 1980s.
The Lighthill report, commissioned by the UK Parliament in 1973, further contributed to the AI winter by criticizing the failure of AI to achieve its “grandiose objectives” and highlighting the problem of combinatorial explosion. This report led to a significant reduction in AI research funding in the UK. In the US, the Mansfield Amendment of 1969 required DARPA to fund mission-oriented research rather than basic undirected research, leading to a more stringent evaluation of AI research proposals. The unrealistic promises made by AI researchers to secure funding, coupled with the disappointment in projects like the Speech Understanding Research program at Carnegie Mellon University, led to a substantial decrease in AI funding by 1974, marking the onset of the first AI winter.
Disillusionment set in during the late 1970s as it became clear the required computing power and fundamental breakthroughs were still lacking. As a result, AI lost its luster with governments and businesses drastically reducing investments. This ushered in an “AI winter” that would last for nearly two decades.
The Second AI Winter
The next AI spring bloomed in the 1980s with a renaissance around expert systems that showed commercial potential by codifying human expertise into rules-based decision models. Once again, the hype spiraled with unrealistic predictions of sentient machines on the horizon.
During the 1980s, a type of AI program called an “expert system” gained widespread adoption by corporations worldwide. Expert systems were designed to emulate the decision-making ability of a human expert within a specific domain. The first commercial expert system, XCON, developed at Carnegie Mellon for Digital Equipment Corporation, was a huge success, estimated to have saved the company $40 million over just six years of operation. Inspired by this success, corporations around the world began developing and deploying their own expert systems. By 1985, companies were spending over a billion dollars on AI, mostly on in-house AI departments. An entire industry grew up to support the development of expert systems, including software companies like Teknowledge and Intellicorp, and hardware companies like Symbolics and LISP Machines Inc., which built specialized computers optimized for AI research.
However, by the early 1990s, the earliest successful expert systems, such as XCON, proved too expensive to maintain, were difficult to update, and could not learn. They also suffered from brittleness, meaning they could make grotesque mistakes when given unusual inputs, and fell prey to problems like the qualification problem, which had been identified years earlier in research on nonmonotonic logic. Expert systems were found to be useful only in a few special contexts, leading to a decline in their adoption.
The end of the Japanese Fifth Generation computer project in 1992 and the cutbacks in the Strategic Computing Initiative (SCI) by DARPA in the late 1980s further contributed to the AI winter. The Fifth Generation project, which aimed to create machines capable of reasoning like humans, failed to meet its ambitious goals. Similarly, under the leadership of Jack Schwarz, DARPA cut funding to AI projects, dismissing expert systems as “clever programming” and focusing on technologies that showed more promise. The combination of these factors led to a significant reduction in AI funding and research during the late 1980s and early 1990s.
The consequences were severe, with major corporations being forced to shutter their AI divisions and research at academic institutions grinding to a halt in what became known as the “second AI winter.” This period of retrenchment lasted until the late 1990s and early 2000s when statistical machine learning techniques powered by increased data and computing resources catalyzed the AI renaissance we’re experiencing today.
The Hype Cycle
The key lesson from these past hype cycles is the critical importance of calibrating expectations and acknowledging the limitations of current AI systems. Overly bullish predictions from researchers, amplified by sensationalistic media narratives, set the AI field up for inevitable disappointment and backlash when the promised accomplishments inevitably fell short.
Moreover, the lack of key enablers like sufficiently big data, powerful hardware, and theoretical breakthroughs ultimately capped the progress that could be made during the initial waves of narrow, rules-based AI development. Only once those constraints were overcome did today’s transformative techniques like deep learning become viable.
So, while the current AI spring has a decidedly different character, stemming from fundamentally more capable approaches, it would be naive to assume the entire field is immune to another precipitous fall from grace. Just as past eras have demonstrated, public image and institutional commitment can prove fickle if excessive hype and optimism aren’t counterbalanced with objectivity about AI’s genuine frontiers and readiness.
For sustained progress in AI development and adoption, all stakeholders – from researchers and engineers to business leaders and policymakers – must strike a delicate balance. On one hand, limitless ambition, imagination, and confidence in AI’s long-term potential is vital to inspiring fundamental research and catalyzing innovation. Visions of advanced AI assistants, autonomous vehicles, personalized healthcare, and more should indeed drive us forward.
At the same time, experts must take great care to shape the public narrative around AI’s real-world readiness in the near and medium term. Acknowledging limitations, governing risks like secure and unbiased systems, and emphasizing current AI’s status as incredibly powerful but narrow tools is crucial. Failing to do so could foment another wintertime backlash as these systems begin proliferating into mission-critical applications and user experiences that don’t match the utopian hype.
We are close to the peak of the hype cycle, with widespread belief that generative AI techniques will replace authors, musicians, actors, customer service associates, developers, among a wide range of other careers and industries, within the next decade.
However, there are real concerns whether the pace of development can be maintained due to the data-hungry nature of modern AI algorithms, and we may be running out of high-quality training data for AI models. Furthermore, modern LLMs (such as ChatGPT) are each plagued by the same major issue with no clear solution in sight: hallucinations. “Hallucinations” are a tendency for LLMs to generate nonsensical or factually inaccurate information. This effect is bound to occur due to the probabilistic nature of Transformer architecture which underlies modern LLMs and will never be entire eliminated if they are based on neural network models.
Finally, copyright litigations could yield a massive blow to generative AI and its development. There are currently at least 8 major copyright lawsuits brought against OpenAI, Microsoft, Github, and other providers of generative AI tools claiming that copyrighted material was utilized for training AI models without consent. There has been much analysis already written about these lawsuits, suffice it to say that a major ruling in any of these cases could add a huge cost to the development of any new language models.
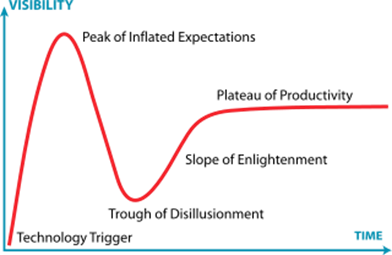
We are here.
As such, it is more important than ever to take each astonishing and breathless announcement with a grain of salt, and remember we are in a hype cycle. The advancements in AI will undoubtedly have a major impact in the years to come, but just like the internet and the dotcom crash, some things take time to mature so we can understand their full value. By responsibly educating the public and key decision-makers about the real capabilities and limitations, the AI community can position itself for stable, long-term progress. Learning from the cycles of enthusiasm and disillusionment that have plagued AI’s past, today’s pioneers have an obligation and opportunity to get it right. Doing so will usher in an enduring season of AI innovation and impact.
Visit here to learn more about AI Solution Use Cases